Unlocking the Power of Labeled Image Datasets for Business Growth
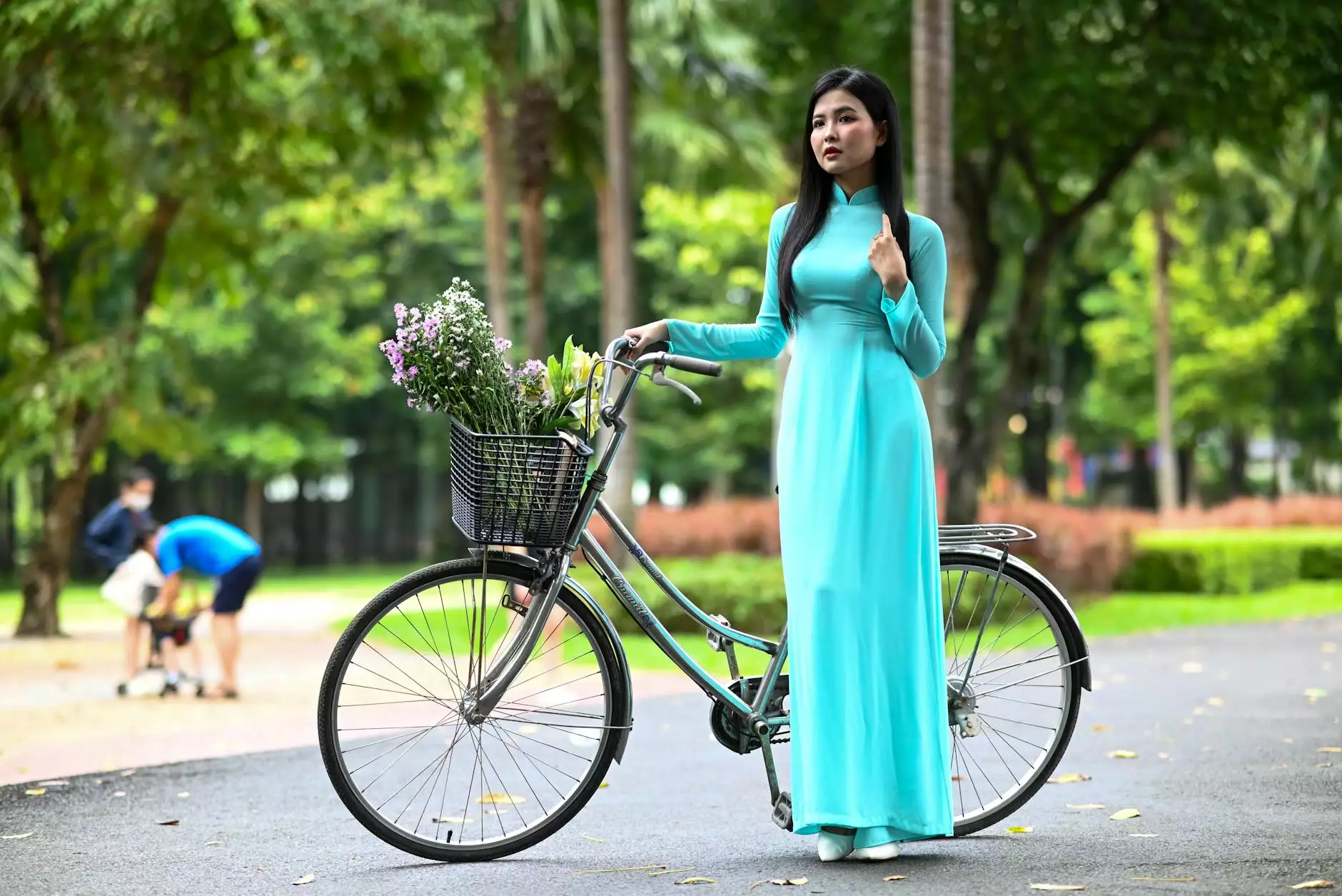
The Significance of Labeled Image Datasets in Today's Digital Landscape
In the era of rapid technological advancements, businesses are increasingly relying on labeled image datasets to enhance their operations. These datasets serve as the backbone for artificial intelligence (AI) and machine learning models, enabling companies to interpret, analyze, and utilize image data effectively. As organizations strive for digital transformation, understanding the value of these structured datasets is crucial.
What Are Labeled Image Datasets?
Labeled image datasets consist of images that have been annotated with descriptive labels. These labels outline specific characteristics or features within the images, providing context that is essential for training AI models. For instance, in the field of computer vision, labeled datasets help in recognizing objects, faces, and even complex scenes within an image.
The process of labeling can involve defining boundaries around objects, identifying attributes, or providing text descriptions. This meticulous effort significantly enhances the reliability and accuracy of AI predictions, ultimately leading to better decision-making in businesses.
Importance of Data Annotation Tools and Platforms
To leverage the potential of labeled image datasets, organizations must invest in robust data annotation tools and platforms. These tools automate the labeling process, enabling teams to handle large volumes of image data efficiently. Such tools not only enhance productivity but also maintain high standards of accuracy.
The integration of automation in data annotation minimizes human error while managing intricate tasks, making it indispensable for businesses aiming for scalability and consistency. Here are some key features that a top-tier data annotation tool should possess:
- User-Friendly Interface: A straightforward design allows users to focus on annotating rather than navigating complex software.
- Collaboration Capabilities: Features that support teamwork enhance productivity, allowing multiple users to annotate simultaneously.
- Advanced Annotation Techniques: Tools that offer polygonal, line, and box annotations enable precise labeling of intricate images.
- Quality Control Mechanisms: Automated reviews and validation processes help maintain high annotation quality.
The Role of Labeled Image Datasets in AI and Machine Learning
The relationship between labeled image datasets and the development of AI and machine learning models is pivotal. These datasets serve as both the training ground and the evaluation set for models. When training a model, the data must be properly annotated to teach the system how to understand and predict outcomes based on new, unseen images.
Here’s how labeled image datasets impact AI and machine learning:
- Training Efficiency: A well-annotated dataset accelerates the learning process by providing clear examples for the model to learn from.
- Improved Accuracy: Accurate labels lead to better predictions. A model trained on quality datasets performs significantly better when applied to real-world tasks.
- Task Specialization: Different industries can create specialized datasets for tasks such as medical image analysis, autonomous driving, and facial recognition, tailoring AI development to their specific needs.
- Continuous Learning: Labeled datasets can be used to refine models over time, allowing them to adapt to new data and trends.
Benefits of Using Labeled Image Datasets in Business
Leveraging labeled image datasets provides numerous advantages for businesses across various sectors. The following are some ways businesses can benefit:
- Enhanced Customer Experience: AI applications that utilize labeled datasets, such as personalized image recommendations and chatbots, can dramatically improve user engagement and satisfaction.
- Operational Efficiency: Automation of tasks like image classification and monitoring allows for faster decision-making and reduced operational costs.
- Innovation in Products and Services: Companies can develop new offerings based on insights derived from image data, opening up fresh revenue streams.
- Competitive Advantage: Organizations that adopt data-driven strategies using labeled datasets can outperform competitors who rely on conventional methods.
Case Studies of Successful Implementation
Numerous businesses have harnessed the power of labeled image datasets to achieve remarkable success. Here are a few noteworthy examples:
1. HealthTech Innovations
A healthcare startup utilized labeled datasets for medical imaging analysis. By annotating thousands of radiological images, the company trained AI algorithms to detect anomalies in X-rays and MRIs, significantly reducing diagnosis time and improving patient outcomes.
2. Retail Sector Enhancement
An e-commerce giant employed labeled images to enhance product search features. By categorizing products through detailed annotations, customers could find items more efficiently, leading to a remarkable increase in sales conversions.
3. Autonomous Vehicles
The automotive industry invests heavily in labeled image datasets for autonomous vehicle development. By training machines with millions of labeled images of road scenarios, companies are capable of creating systems that enhance safety and reliability in self-driving technologies.
Challenges in Working with Labeled Image Datasets
Despite the many benefits, creating and maintaining labeled image datasets comes with challenges:
- Cost and Time: Manual annotation can be resource-intensive, requiring significant time and financial investment.
- Quality Assurance: Ensuring high-quality annotations is crucial, as poor labeling can lead to misinterpretation by AI systems.
- Scalability: As the volume of data increases, scaling annotation efforts can be a daunting task for many organizations.
Overcoming the Challenges of Data Annotation
To mitigate these challenges, organizations can adopt various strategies:
- Invest in Automation: Leveraging advanced tools that use AI to assist in the annotation process can substantially reduce time and costs.
- Utilize External Annotation Services: Companies can outsource data annotation to specialized service providers, allowing them to focus on core tasks.
- Implement Strong QA Practices: Regular audits and validations of annotated datasets ensure consistent quality and accuracy.
Future Trends in Labeled Image Datasets
As the digital landscape continues to evolve, so do the methods and applications of labeled image datasets. Predictions for the future include:
- Increased Utilization of AI for Annotation: AI-driven annotation tools will improve efficiency and accuracy.
- Real-Time Annotation Platforms: Innovations in cloud technology will allow real-time collaboration on datasets, making the annotation process fast and dynamic.
- Expanding Fields of Application: New industries such as agriculture and environmental monitoring will increasingly rely on labeled image datasets to drive decision-making.
Conclusion: Embracing Labeled Image Datasets for Future Success
In conclusion, the importance of labeled image datasets cannot be overstated in today's data-driven business environment. By investing in quality data annotation tools and platforms, organizations can unlock the full potential of their data. The ability to transform images into actionable insights is a game-changer for businesses aiming to stay competitive and innovate within their industries.
As we look toward the future, embracing labeled image datasets will be key to crafting intelligent systems that power every facet of business operations. The journey ahead is paved with opportunities for growth, efficiency, and enhanced customer experiences, all stemming from the effective use of image data and its annotations.